AI for Analytics: How Augmented Analytics is Transforming BI

.png)
AI is fundamentally reshaping the business intelligence (BI) and analytics landscape.
What once took hours of manual data wrangling, slicing/dicing, and insights generation is now possible in seconds or minutes—no SQL/Python/Excel necessary—and more people than ever are getting answers to what is happening, why things are changing, and what to do next, thanks to AI augmented analytics. If you aren't exploring how AI can augment analytics, your firm runs the risk of falling increasingly behind in this rapidly evolving AI landscape.
But don’t fear! This post will get you up to speed on AI augmented analytics—including how cutting edge technologies like AI Agents and agentic flows are augmenting augmented analytics—so you can turn these powerful tools into your advantage, leapfrogging legacy approaches (and your competition!) for better/faster decisioning. Let’s dive in.
What Is Augmented Analytics?
Augmented analytics is a dramatically faster way to turn data into actionable insights using AI such as machine learning (ML), natural language processing (NLP), generative AI (GenAI), and increasingly, agentic AI.
Augmented analytics unlocks rapid, robust data-driven decisioning for all by:
- Democratizing Analytics: No-code conversational interfaces allow anyone—regardless of technical or analytical experience—to ask and answer questions ranging from quick ad-hoc to complex business-specific questions without the need for time consuming dashboards, waiting in analytis ques, or manually pulling data from various sources and pivoting it.
- Expediting Analysis: Intelligent automation is embedded throughout the entire data to insights workflow, allowing users to quickly get answers and insights and/or setup their own agentic analytics workflows
- Deeper Insights: AI Insights and multistep agentic analytics flows parse billions of variables to get to the true drivers and root causes behind metric changes
.png)
What Makes it ‘Augmented’ vs Traditional BI?
Augmented analytics differs from traditional BI analytics in several key regards:
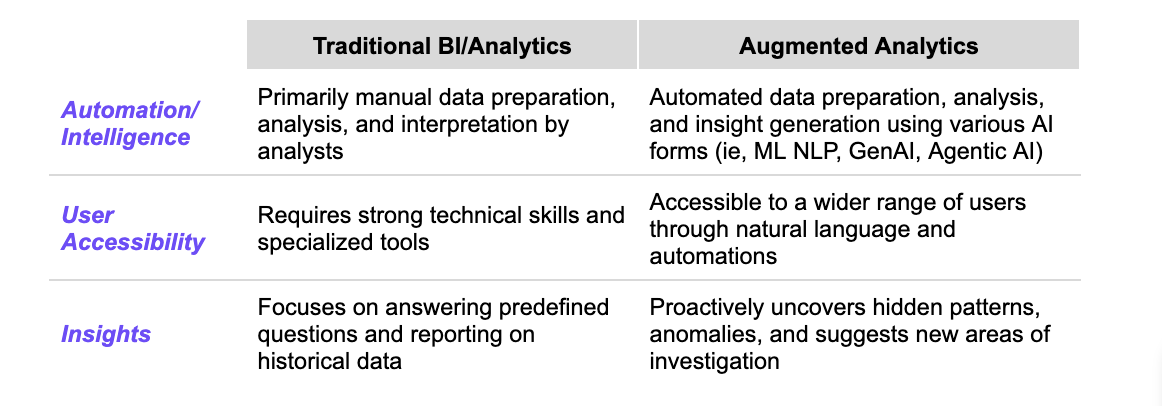
Augmented analytics represents a fundamental difference in approach compared to traditional tools. Legacy BI platforms struggle to deliver on the promise of true self-service analytics because:
- Traditional tools weren't built for the complexity or speed modern businesses demand. They were built for a world where data lived in a warehouse, questions were static, and insights could wait a week. That world no longer exists. Today, data is scattered across clouds, apps, and pipelines. Questions change daily. And decisions can't wait. Yet many analytics platforms still operate on a patchwork of rigid dashboards, manual prep steps, and bolt-on AI features that weren't designed to work together.
- The Architectural Gap. Legacy tools rely on static dashboards and slow data pipelines. They separate data prep from insight generation from data catalog from advanced analytics, compelling users to jump between tools and teams. Even those with AI capabilities often treat them as surface-level add-ons, limited to chart summaries or natural language queries. That means:
- Answers take days or weeks.
- Most users are stuck waiting on analysts.
- Opportunities get missed because no one has time to dig deeper.
Evolution of BI & Analytics Culminating in the Augmented Era
To better understand what best-in-class augmented analytics looks like and where it's going, lets quickly look at where it came from.
There have been 3 key waves of BI/analytics, with AI augmented analytics as the latest:
.png)
- Wave 1: IT-Led. IT-led BI/analytics platforms were largely only accessible to a handful of technical people (i.e., low org adoption) and took days/weeks to get an insight (i.e., slow time to insight).
- Wave 2: Analyst-Led. Next came the analyst-led analytics wave as a reaction to the IT-led one with the goal of greater flexibility and end user access. Visualization-first BI platforms like PowerBI and Tableau popularized dashboarding, improving analytics accessibility to analysts. However these platforms fell short of achieving true self-service analytics—whereby anyone could answer any question on their own—because they were still focused on manual descriptive analytics (e.g. ‘what happened’), requiring onerous processes to get to the ‘why’ and ‘what next’.
- Wave 3: AI-Led. Today we are in the AI augmented analytics wave. Led by firms like Tellius, this modern approach leverages ML, NLP, GenAI, and increasingly Agentic AI to dramatically expedite and improve analysis. Within augmented analytics, an evolution has occurred:
- Search-based Analytics. Early augmented analytics vendors offered keyword-based, text-to-SQL data querying capabilities for business users to ask things like “show me monthly revenue" and get a visualized answer. While this was an improvement over static dashboards, it still required SQL-like query syntax and extensive data modeling to ensure these ad-hoc searches functioned properly.
- AI Analytics: Next gen augmented analytics vendors built upon Search by adding machine learning (ML)-powered diagnostic analytics, offering users no-code ways of identifying trends, correlations, anomalies and more. AI Analytics tools also leveraged AI augmentation for more than just exploration, for example, by introducing AI-augmented data modeling, data preparation, predictive modeling, and more.
- GenAI Analytics: Soon after ChatGPT debuted, GenAI-based analytics approaches emerged as new layers to existing AI Analytics platforms and as standalone LLM-powered text-to-SQL startups. GenAI analytics improved upon Search-led Analytics by offering truer natural language query and generative AI-based narrative summaries, bringing fluent explanations and dialogue-like interactions. According to a McKinsey report, GenAI could automate up to 60%–70% of data processing and decision-making tasks in knowledge work.
- Agentic Analytics: The bleeding edge of augmented analytics involves GenAI-powered AI agents that understand a user’s business and analytics goals, plan an approach to derive insights, then run multi-step analyses and adapt in real time like a virtual analyst embedded in your workflow. For example, a sales leader cause ask, "Show me how to reallocate the budget to close Q4 gaps" and an agent ingests sales pipeline, territory, and campaign data, evaluates performance across regions, and recommends budget shifts based on likely return without involving five teams or a week of prep.
Now that we understand the history and progression of business intelligence, let's look at the technology and capabilities that underlie best-in-class augmented analytics platforms.
How Augmented Analytics Works
Augmented analytics transforms raw data into insights by intelligently assisting users join, clean, explore, spot drivers, share, and operationalize data.
.png)
- Augmented Data Connectivity & Preparation. AI transforms data preparation by auto-detecting join keys, suggesting cleansing operations, and resolving terminology conflicts across departments. Live connectivity across cloud platforms eliminates duplications and reduces preparation time by up to 80%.
- Natural Language Data Exploration. Users can ask business questions through conversational interfaces rather than building charts manually. The system breaks down complex queries, identifies patterns, and delivers insights in plain language, often within seconds.
- Automated Insight Generation. AI agents convert complex analytical needs into simple goal statements and chain multiple analyses together automatically. This orchestration saves days of manual effort when addressing multifaceted business questions.
- Advanced Analysis Capabilities. Includes AutoML and explainability features that enhance analytical depth.
- Sharing & Actions. GenAI creates customized summaries and visualizations focused on what changed, why it changed, and recommended actions. Proactive alerts notify teams when metrics shift, enabling early intervention.
These capabilities transform analytics from a support function into a strategic driver of business growth.
The Role of AI in Augmented Analytics
Various forms of AI are leveraged within augmented analytics:
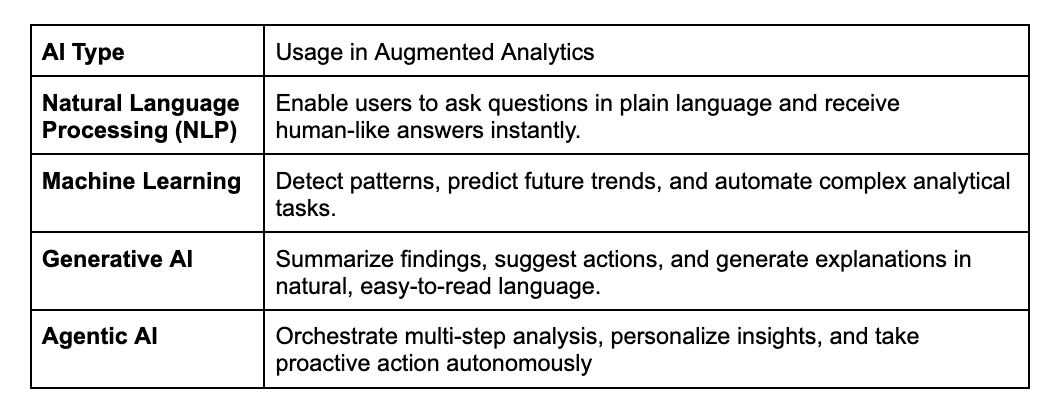
What Are the Benefits of Augmented Analytics?
The true power of augmented analytics isn't just in automation; it's in what enables your teams to do more often, more confidently, and with far less effort. When AI agents and GenAI workflows take over the heavy lifting, analytics stops being a bottleneck and starts becoming a daily advantage.
Here are the core benefits:
.png)
1. Transforming Impossible Insights into Daily Deliveries
Many of the most valuable business analysis tasks are too time-consuming to perform regularly. Consider a global pharmaceutical company seeking to identify high-prescribing doctors it overlooked in last year's outreach. The process usually means pulling data from five systems, merging Excel files, and running manual lookups, a project that takes days.
With agentic analytics, a business user can simply ask: "Which high-prescribing doctors didn't we engage last year but should have?" An AI agent pulls the data, joins it, runs cohort and key driver analysis, and delivers the result in minutes. Tasks that were once too tedious to repeat become a routine capability.
2. Faster, Deeper, More Usable Insights
Augmented analytics platforms analyze billions of records in seconds, detect hidden segments, identify anomalies, and explain the why behind changes, not just the what. Users get real-time answers in natural language, enriched with charts and narrative summaries. This reduces time-to-insight by up to 60%, helping teams react quickly to emerging opportunities and risks.
3. AI Agents Transform Time-consuming Multi-step Analysis
AI agents and agentic flows allow you to perform time-consuming multistep analyses that you typically only do once a month or quarterly. These valuable analyses, such as identifying top-prescribing healthcare providers from last year that haven't been contacted, often require navigating multiple systems and manually processing data. With agentic analytics, these become daily or weekly capabilities rather than occasional projects, unlocking white space opportunities by enabling analysis that simply wasn't getting done before.
4. Higher Adoption Across Business Teams
Augmented analytics is no longer reserved for analysts since everything runs on plain language and intuitive visual outputs. Whether it's a supply chain manager asking about delayed shipments or a marketer checking campaign ROI, anyone can explore data without writing a single formula or SQL query. This shift boosts BI adoption from 30% to over 50%, increasing the value of your data stack.
5. Cross-functional Collaboration and Shared Context
In traditional BI, insights often stay locked within teams. Marketing may have one view of customer churn, while support has another, and they rarely align. Augmented analytics breaks this siloed model. Because queries pull from unified datasets and outputs are easily shareable, teams operate from the same facts, not fragmented reports.
6. From Knowing What Happened to Deciding What to Do
Most BI tools stop at visualization. Augmented analytics go further, suggesting next-best actions and generating reports tailored for decision-making. Executive teams don't just get a chart; they get a story, a recommendation, and a reason. And because agentic flows can repeat this process endlessly, it becomes part of your company's everyday decision rhythm, not a quarterly deep dive.
Now let's examine some real-life examples of augmented analytics in practice.
Augmented Analytics Use Cases
Augmented analytics is transforming decision-making across various industries by enabling faster, data-driven insights. Here are some real-world examples of how organizations are addressing critical challenges:
Pharmaceutical and Life Sciences: Enhancing Market Access and HCP Engagement
A global life sciences organization faced challenges in developing data-driven targeting methodologies for healthcare professionals (HCPs). Using augmented analytics, the market access team achieved a 66% growth in patient starts without expanding the team, resulting in $1.6 million in resource savings. The team can now iterate in real time with the business, leading to improved decision-making across brands.
Financial Services: Accelerating Credit Risk Analysis
A top 10 financial services firm sought to enhance its credit risk analysis capabilities. Utilizing automated machine learning (Auto ML), the firm can now classify segments of customers who are at a higher risk of default. This approach helped the firm save thousands of hours in productivity and over $700,000 in mitigated credit losses per month.
Consumer Packaged Goods: Proactive IT Operations Monitoring
A Fortune 100 consumer goods company’s IT operations team supports over 8,000 software applications. By adopting augmented analytics, the team transitioned from reactive to proactive monitoring, reducing application risk assessment time from five days to mere hours. This efficiency gain resulted in savings of $6 million to $10 million in full-time equivalent costs across various roles.
Retail: Gaining Shopper Insights for Personalized Marketing
Shopper insights teams at modern consumer packaged goods organizations face challenges with the increasing size and complexity of data. By leveraging augmented analytics, these teams can combine sales data with syndicated data from providers like Nielsen and IRI, enabling quick ad hoc data analysis. This approach provides a comprehensive view of consumer behavior, enabling the creation of detailed shopper profiles and driving more effective marketing campaigns.
Here are some additional use cases:
.png)
These examples demonstrate the tangible benefits of implementing augmented analytics, showcasing improvements in efficiency, decision-making, and financial outcomes across various sectors.
How Tellius AI Delivers Augmented Analytics for Modern Businesses
Several platforms claim to support augmented analytics but Tellius AI stands out because of our unified, agentic experience that combines AI-powered automation, natural language interaction, and deep decision intelligence in one place, which accelerates time-to-insight and makes high-value analysis routine, not rare:
1. Augmented Data Connectivity, Preparation, & Modeling
Tellius simplifies one of the most time-consuming parts of analytics: getting data ready to analyze.
- GenAI and AI agents automatically recommend joins, detect data quality issues, and suggest transformations, reducing prep time by up to 80%.
- The platform includes a unified semantic layer that infers data models and understands business context across teams.
- With live connectivity to leading cloud data sources, there's no need for data duplication or movement.
- Users can build pipelines with no-code, low-code, or full-code, giving flexibility to everyone from business users to data engineers.
2. Conversational, Ad Hoc Analysis
Once connected to data, business users don't need to learn SQL or dashboard tools to ask questions in Tellius.
- The platform offers a ChatGPT-like interface that supports natural language questions and follow-ups.
- Agentic flows turn complex, multistep questions into single queries, with the system handling the orchestration.
- Visual outputs are clean, interactive, and instantly shareable via dashboards, embeds, or app integrations.
- Narrative summaries are automatically generated at both the chart and dashboard levels.
3. Augmented Insights and Proactive Intelligence
Once your data's ready, Tellius delivers more than charts. It explains why things happen.
- Automated insight types include key drivers, trend shifts, cohort comparisons, and anomaly detection.
- Each insight is paired with narrative summaries, making complex analysis easy to understand and present.
- Intelligent alerting triggers notifications when metrics shift, highlighting issues you may not have anticipated.
- Insights are dynamically chosen based on data patterns, eliminating the need to configure every scenario manually.
4. AI Agents and Agentic Flows
This is where Tellius leads the next frontier of augmented analytics.
- Users can call AI agents conversationally and launch pre-built or custom analysis flows in seconds.
- The Agent Library includes dozens of ready-to-use templates across departments and industries.
- The Agent Composer empowers users to create no-code multistep flows, chaining together data pulls, segment analysis, and recommendation generation.
- AI agents don't just analyze, they perceive, reason, and act, turning everyday users into decision-makers.
5. Advanced Analysis for Strategic Decisions
Beyond reporting, Tellius supports advanced decision-making techniques without complexity.
- It includes built-in support for predictive modeling, what-if analysis, scenario planning, and optimization.
- Every model offers row-level explainability, so users can trust and interpret predictions.
- Outputs are easy to export, visualize, and embed directly into decision workflows.
- Whether you're forecasting revenue, prioritizing customer outreach, or modeling supply chain changes, Tellius makes it simple.
6. Monitoring, Learning, and Governance
Tellius ensures that insights aren't just fast; they're traceable, explainable, and continuously improving.
- Users can trace every insight back to its source data, transformations, and even the LLM or SQL logic used.
- Built-in feedback mechanisms allow for real-time refinement of results.
- Learnings are captured at the user, system, and business-view levels, improving personalization over time.
- Underneath it all is a growing knowledge graph, shaping every future interaction with richer context.
How Tellius is Different
Tellius was designed from the ground up for speed, autonomy, and depth.
- It handles billions of records and scales elastically to meet your needs.
- It connects live to your data sources without needing movement or duplication.
- The semantic layer bridges business context across teams.
- GenAI and AI agents aren't stitched on, but imbued into every stage of the workflow: prep, insight, and action.
- AI agents do the heavy lifting of multistep analytics processes, transforming complex queries into simple conversations.
- Agentic flows can run multi-step analyses in minutes, automatically. These agentic decision flows not only analyze the data but also determine the best path forward, helping users move from questions to decisions without delay.
- Every insight is explainable, traceable, and personalized to the user's context.
Instead of building a prettier dashboard, Tellius reimagined the entire pipeline so that analytics can finally keep pace with the business. The architectural advantages of augmented analytics platforms like Tellius translate directly into real-world business outcomes.
Measuring Success with Tellius AI’s Augmented Analytics
Augmented analytics isn't just a technology shift; it's a measurable performance upgrade. Teams using Tellius consistently report faster decisions, higher platform adoption, and deeper business impact. Here's what success looks like when insight becomes continuous:
1. 60% Reduction in Time-to-insight
With natural language querying and agentic flows, Tellius helps teams move from question to answer in minutes, not days. From sales to supply chain, users can ask follow-up questions, drill down instantly, and iterate in real time without analyst bottlenecks.
2. 80% Reduction in Data Preparation Effort
Tellius automates tedious prep work with GenAI-driven joins, cleansing suggestions, and transformation logic. Teams spend less time wrangling data and more time analyzing it, accelerating workflows across functions.
Explore augmented data prep →
3. Higher Adoption Across Business
Tellius eliminates the friction of traditional BI. No SQL. No waiting on custom dashboards. Just ask a question and get an answer. Organizations adopting natural language interfaces and agentic flows have reported analytics usage growing from ~30% to over 50% across teams.
See how shopper insights teams use it →
4. High-value Analysis Becomes Routine
Before Tellius, complex questions such as identifying missed HCPs, segmenting credit risk, or modeling budget reallocations were delayed or skipped due to manual effort. With Tellius, these become part of the daily cycle. Teams shift from reactive indicators to proactive insights.
5. Tangible Impact Across Sectors
While specific results vary, Tellius customers have achieved:
- Faster risk prediction and over $700K/month in avoided losses.
- 5-day IT reporting cycles reduced to hours, unlocking $6M–$10M in team efficiency.
- 66% growth in patient starts and $1.6M saved in resource costs for market access teams
As these metrics demonstrate, augmented analytics represents more than an incremental improvement in business intelligence. It's a fundamental shift in how organizations can leverage their data.
From Insight Lag to Insight Leadership: The Augmented Analytics Advantage
Business teams don't suffer from a lack of data. They suffer from a lack of time, clarity, and execution. Dashboards indicate what happened. Some tools even explain why. But few platforms help you decide what to do and then do it faster than your competition. That's the promise of augmented analytics. With agentic flows, natural language interfaces, and AI-driven automation integrated at every stage, that promise is now a reality.
Tellius gives you more than answers. It gives you momentum. From pharmaceutical teams uncovering missed market opportunities to financial institutions reducing risk in real time, leading organizations are using augmented analytics to move faster, think smarter, and act with confidence.
If your current analytics stack makes you wait, guess, or rely on a small group of experts, it's time to move beyond insight lag and into a world where anyone can ask a question and immediately act on the answer.
Want to see how Tellius helps your team move from data to action faster?
Explore the platform →
Book a demo →
Get release updates delivered straight to your inbox.
No spam—we hate it as much as you do!
Related blog posts
Tool and strategies modern teams need to help their companies grow.

Augmented Analytics: How AI is Transforming BI to Keep Pace With Complex Data
Augmented analytics is transforming business intelligence (BI) by combining artificial intelligence (AI) and machine learning to automate complex data analysis tasks. This innovation empowers businesses to quickly uncover deeper insights, simplify data exploration, and accelerate decision-making, making advanced analytics accessible to all users, regardless of technical expertise.
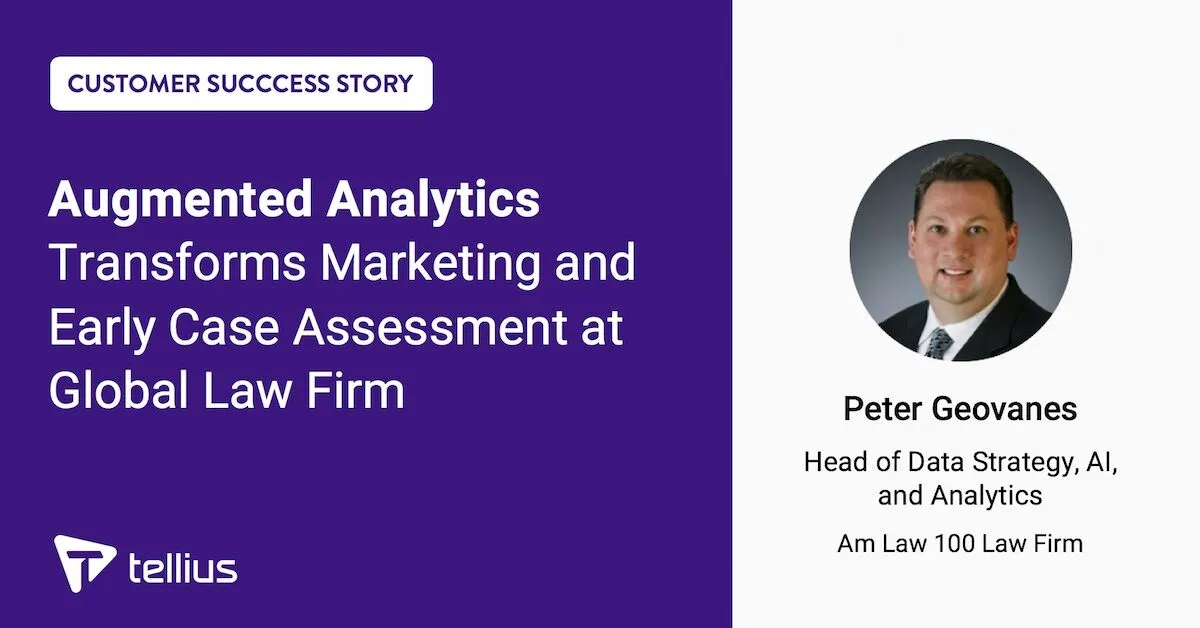
Augmented Analytics Transforms Marketing and Early Case Assessment at a Global Law Firm
Read how augmented analytics helps a top global law firm gain a competitive advantage by creating a data-driven culture.
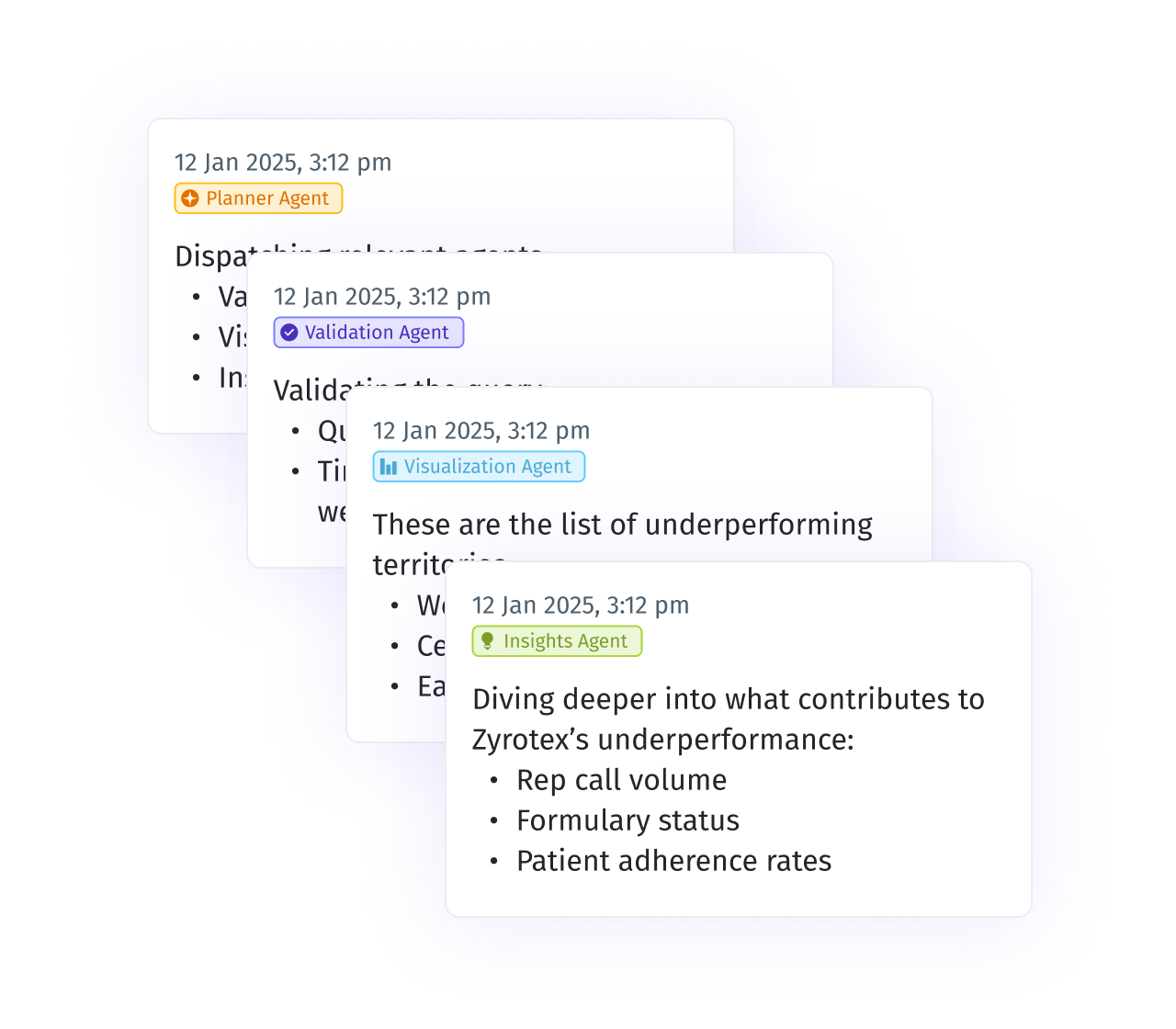
Introducing Tellius AI Agents
Tellius AI Agents and AgentComposer transform business analytics by automating complex multi-step analysis through specialized autonomous agents. Unlike generic chatbots or RPA tools, these agents leverage your enterprise data and business context to deliver deep insights across sales, marketing, finance, and manufacturing—turning questions into actions in minutes instead of days. With no-code agentic workflows, organizations can achieve 100X productivity gains and continuous, data-driven decision making.