How AI Agents Are Finally Delivering on the Promise of Self-Service Analytics

%20(1).png)
Self-service analytics was never really self-service. Until now.
That’s the inconvenient truth most BI platforms won’t say out loud. The promise was simple: empower business users to answer their own data questions without waiting on the data team. But reality hasn’t lived up to the promise.
Dashboard sprawl. Analytics queues. "Self-service" that still requires SQL or an analyst intervention. This isn’t autonomy or self-service—it was a prettier front-end on a backend bottleneck.
Today, AI agents are rewriting that narrative. By combining conversational interfaces with autonomous, memory-rich, and proactive intelligence, agentic analytics finally delivers what self-service always promised: speed, simplicity, and independence. And the shift is already happening.
What Is Self-Service Analytics?
Self-service analytics is the ability for non-technical teams like sales, marketing, operations, and finance to access and analyze data on their own, without relying on data engineers or analysts.
.png)
A Brief History of Self-Service Analytics
The concept gained traction in the early 2010s as organizations looked to scale insights beyond data teams. Tools like Tableau and Qlik promised to "democratize data" with drag-and-drop interfaces. Later, Power BI and Looker made similar promises with embedded dashboards and role-based views.
%20(1).png)
But over time, cracks formed:
- Tools got more powerful, but also more complex.
- Dashboards proliferated, but were disconnected from decisions.
- Users were overwhelmed, not empowered.
The result? Most business users lacked the ability to get quick answers to “what” is happening or reach the “why” behind business changes without bothering an analyst. “Self-service” became synonymous with surface-level data access, not independent answers & insight generation.
Real transformation required more than just a UI facelift. It required intelligence, automation, and context.
Self-Service Analytics Is Broken (And Everyone Knows It)
%20(1).png)
Let’s face it–self-service analytics is broken:
- Adoption is low. Despite millions spent of self-service tools, analysts are still under water with requests from the business and business users are still waiting days or weeks for answers. Analytics adoption rates hover in the 20% range.
- Data literacy gaps are real. Gartner found 47% of analytics leaders cite data literacy as one of their top three challenges. Most business users aren’t equipped to interpret dashboards, let alone write their own queries.
- Workflows are fragmented. Even if users can ask questions, they still need to stitch together datasets, dig through charts, or escalate to the data team for anything deeper than surface-level findings.
Meanwhile, leaders are under pressure to move faster, act smarter, and make data-driven decisions at scale. The status quo isn't just inefficient—it's unsustainable.
GenAI Was a Step Forward—But Not the Finish Line
Generative AI was a major leap forward for self-service by making querying massive datasets in natural language easier and less formulaic. But a naive implementation of GenAI for analytics—that is, simply slapping an LLM-powered text-to-SQL interface onto traditional BI tools—has some major pitfalls.:
- They’re shallow—they can’t reach the insight depth you need because they don’t understand your specific business metrics, logic, and goals (they lack context and semantics, in other words)
- They hallucinate—they'll provide an answer, even if it's a hallucination
- They aren’t connected to enterprise systems—e.g., they may demo just fine on .CSV files, but when it comes to doing real analysis, they don't handle messy enterprise data well.
That’s not enough.
When business users ask, "Why are sales down in the Northeast and what can I do about it?", they don’t want a chart. They want an answer with context, a root cause, and a next best action how to address the situation.
That’s where AI agents help.
.png)
Why Conversational Is Crucial—But Not Enough
Natural language is the gateway to true self-service, but AI agents are the engine.
A chat interface alone doesn’t make analytics accessible. AI agents make it happen by:
- Maintaining memory across questions
- Understanding business logic (e.g. what "NBRx" means for your brand)
- Orchestrating the right workflow behind the scenes
This blend of conversational UI + agentic execution is the key to true self-service intelligence.
Meet the New Self-Service Model: Agentic Analytics
AI agents don’t just answer questions—they execute multi-step analytical processes on a user’s behalf, starting from a conversation.
Instead of clicking through dashboards or endlessly slicing & dicing data, business users just describe their goal—something like "I want to understand why revenue dropped in Q1" or "Which targets are most likely to convert next month?"—and the agent takes it from there.
This goal-driven, conversational entry point makes insights instantly accessible. It’s not about data access anymore—it’s about business outcomes. And it’s the first time business users can truly self-service the insights they need to drive big decisions.
Think of them as intelligent digital analysts that:
- Connect to multiple datasets
- Understand your business terminology
- Automate exploration, root cause analysis, and recommendations
- Learn and personalize over time
In this model, self-service isn’t a dashboard. It’s a dialogue—guided by agents that do the work.
%20(1).png)
The Missing Piece: A Semantic Knowledge Layer
AI agents are only as smart as the context they understand. That’s why a semantic knowledge layer is foundational.
This layer sits between raw data and the AI agent, providing structure and meaning to business concepts. It knows that "TRx" is total prescriptions, "Tier 1 HCPs" are prioritized targets, and "pull-through" refers to specific post-script activities.
With this layer:
- Agents map natural language to the right logic and data
- Insights are accurate, consistent, and explainable
- Business terms are standardized across users and teams
Without it, you’re right back to chatbots guessing your intent.
%20(1).png)
Real-World Example: Field Sales in Pharma
Let’s look at a commercial pharma use case.
A sales director asks:
“Why did NBRx drop in Q1 for Brand X in the Southeast?”
With traditional tools:
- They’d pull a report (if it exists).
- Manually filter by geography, brand, and date
- Spot the trend, then call in an analyst for root cause analysis
With an AI agent:
- It identifies the relevant datasets (3rd party prescription data, sales call activity, etc.)
- Runs cohort analysis to find underperforming geographies and healthcare professionals (HCPs)
- Surfaces the insight: a key segment of HCPs stopped engaging after a formulary change
- Recommends the next step
The user didn’t click a single dashboard. That’s the new self-service.
What to Look for in an Agentic Self-Service Platform
Here’s your checklist:
- ✅ Natural language querying with business context
- ✅ Deep automated insights
- ✅ Memory across questions and users
- ✅ Auto-orchestration of multi-step analysis
- ✅ Personalization and learning over time
- ✅ Seamless access to cloud data (no extracts)
- ✅ No-code agent building
- ✅ Semantic knowledge layer for consistency and clarity
If a tool can’t do all of this, it’s not self-service. It’s lipstick on SQL.
Tellius is Purpose-Built for Agentic Self-Service
Most BI tools aren’t built for this. They bolt GenAI onto a dashboarding engine.
Tellius is different. It was built for agentic analytics from the ground up:
- Kaiya—Conversational AI with deep semantic understanding and memory. It remembers who you are, what you care about, and can answer your ‘what’, ‘why’, and ‘what-if’ questions seamlessly, in natural language.
- Deep Insights—AI automated root cause analysis, key drivers, cohort comparisons, and anomaly detection with multilevel drills and dynamically generated narratives that turn complex analysis into digestible takeaways
- Agent Composer—A no-code builder to create and customize analytical agents. Anyone can orchestrate complex logic without a line of SQL.
- Learnings—Persistent personalization. Agents adapt to feedback, preferences, and user roles.
- Knowledge Layer—Built-in semantic model of your business that defines KPIs, hierarchies, and business logic so agents can reason like your best analyst.
Together, this stack doesn’t just support self-service. It fulfills it.
TL;DR: Self-Service, Finally Delivered
- Self-service BI and analytics tools failed to deliver independence, speed, and ease
- AI agents change the game—handling complexity, reasoning, and automation behind the scenes
- A semantic knowledge layer ensures agents are accurate and business-aware
- With Tellius, users don’t need to learn analytics. They just need to ask.
Self-service was never really self-service. Until now.
Get release updates delivered straight to your inbox.
No spam—we hate it as much as you do!
Related blog posts
Tool and strategies modern teams need to help their companies grow.
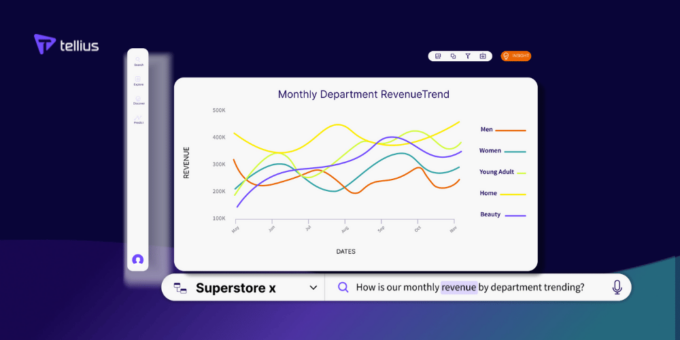
Self-Service Analytics Explained: Benefits, Challenges, and the AI Revolution
Why are more organizations turning toward self-service analytics? Businesses thrive when everyone—not just the data experts—has access to the tools and information they need to make quick, insightful decisions driven by data.
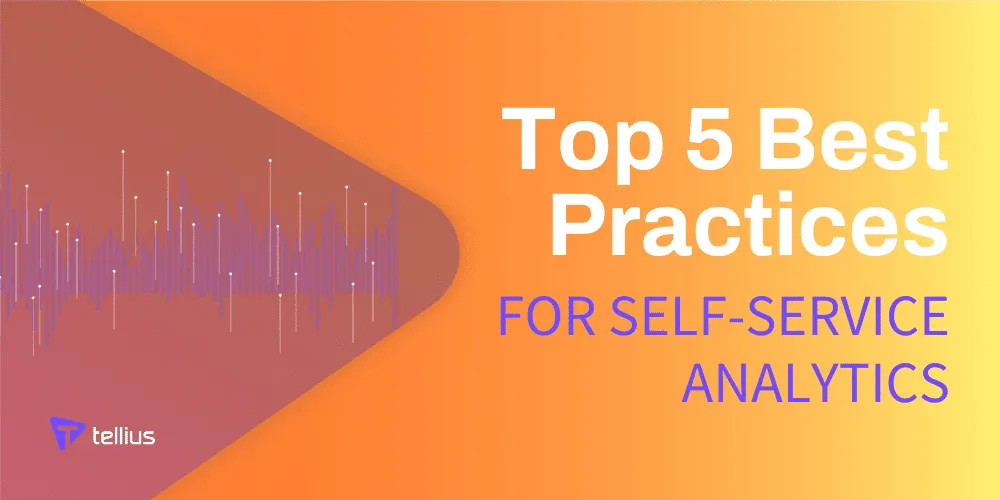
Top 5 Best Practices for Self-Service Analytics
The benefits of self-service analytics are substantial. With a self-serve model, you can start to truly scale your organization’s data-driven decision-making approach.
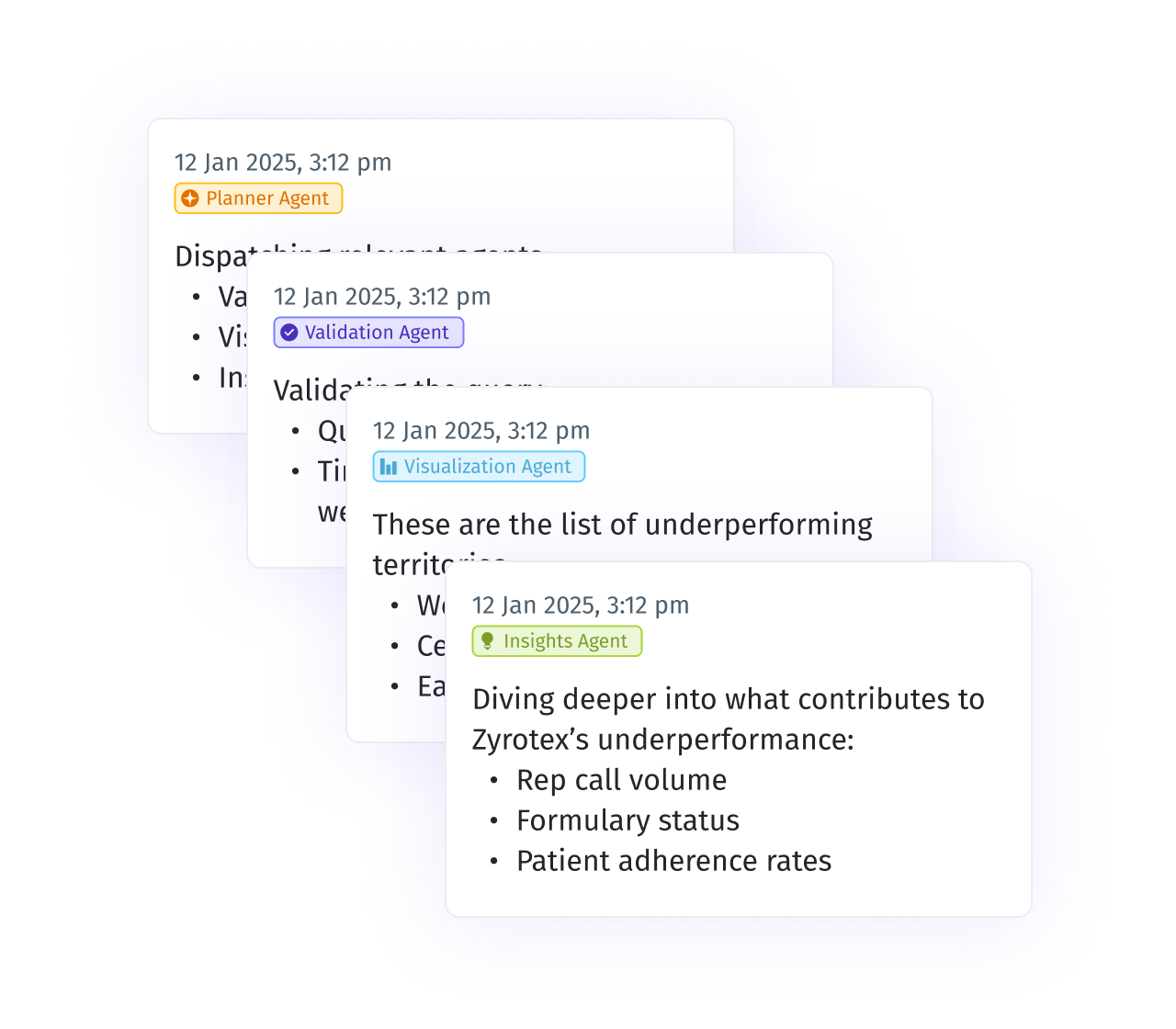
Introducing Tellius AI Agents
Tellius AI Agents and AgentComposer transform business analytics by automating complex multi-step analysis through specialized autonomous agents. Unlike generic chatbots or RPA tools, these agents leverage your enterprise data and business context to deliver deep insights across sales, marketing, finance, and manufacturing—turning questions into actions in minutes instead of days. With no-code agentic workflows, organizations can achieve 100X productivity gains and continuous, data-driven decision making.

Augmented Analytics: How AI is Transforming BI to Keep Pace With Complex Data
Augmented analytics is transforming business intelligence (BI) by combining artificial intelligence (AI) and machine learning to automate complex data analysis tasks. This innovation empowers businesses to quickly uncover deeper insights, simplify data exploration, and accelerate decision-making, making advanced analytics accessible to all users, regardless of technical expertise.