Four reasons data analytics projects fail (Part 1)
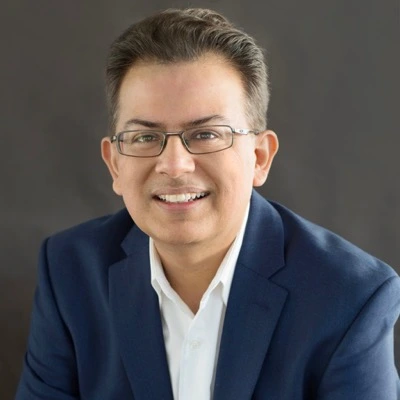
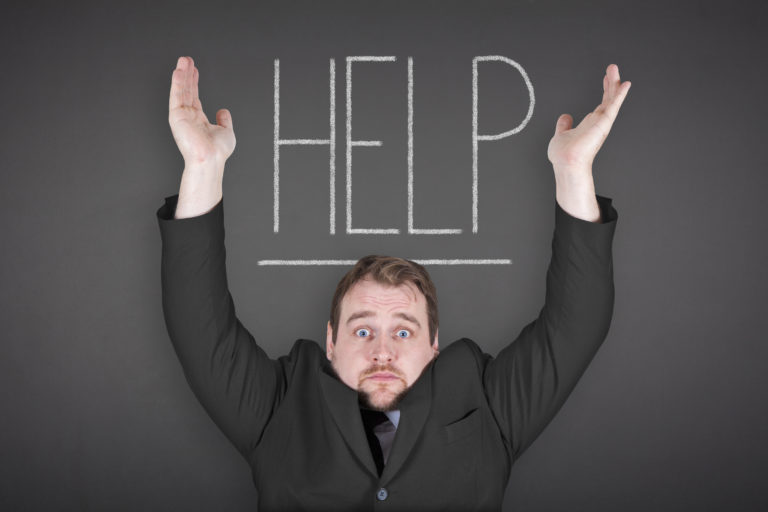
Data analytics projects can be particularly difficult to implement and execute. Not only is the information often hard to ingest, transform and explore, but the support staff needed to implement and maintain traditional analytics projects can be hard to find and keep. This and a myriad factors can lead to less than stellar results from big data undertakings, highlighting the various dependencies that make analytics work.
Research by PwC, found that an alarming number of enterprises still falter out of the starting blocks, suggesting a lack of vision in turning data into a profitable or competitive asset. The report states that data, “…can be bought, sold, shared and even stolen -- all things that suggest that data has value. Yet when we conducted our research very few organizations can attribute a value and, more concerning, many do not yet have the capabilities we would expect to manage, protect and extract that value." While it’s more than evident that big data acts as the fuel for business performance across virtually every aspect, many companies still, sadly, get it wrong. This blog, the first in a series, covers four data analytics challenges companies must overcome to find that sweet spot between analytics and insights.
- IT resistance: From C-Suite executives to seasoned programmers, resistance in IT departments can be one of the major reasons data analytics projects fail. There are often quite a few overheads involved in big data projects and many stakeholders within the IT department may see it as another piece of technology that needs support. Traditional big data systems also involve a steep learning curve for business users, which typically means that IT needs to provide training. The need for self-service and intuitive training has evolved from this learning curve and the training that is traditionally provided by IT. McKinsey puts it this way, “Getting analytics out of the exclusive hands of the statistics gurus, and into the hands of a broad base of frontline users, is seen as a key building block for scale. Without needing to know a single line of coding, frontline users of new technology tools can link data from multiple sources”
- Data silos: In many industries such as healthcare, automotive, engineering, construction and insurance, for example, the organization is siloed, which can severely hamper big data projects because the business structure and culture isn’t really set up for innovation. According to Forrester Research, “Analytics improves an organization’s ability to understand, anticipate, and act on data to drive customer value, business efficiency, and growth. But data is diverse, dispersed across systems, and dynamic — and now includes more and more crowdsourced and social data.” The relationships between datasets, and inherently their fusion, make custom development efforts difficult to execute. Many companies with siloed structures would need more powerful platforms that are data agnostic in order to execute successful big data projects.
- Lack of easy access to business insights: Big data only becomes useful if it is able to answer important questions quickly and intelligently. A manufacturing company, for example, might want to know which products were the most profitable in a specific region over the past quarter, but most platforms can’t interpret questions like this or provide accurate and meaningful insights without calling in a team of data scientists. The process of uncovering business insights is still fairly unsophisticated in the majority of big data platforms, leaving the average business user unable to uncover opportunities that may lay dormant. To make analytics truly work – in a democratized way – analytics platforms need to become more intuitive and far less cryptic, giving the entire business the ability to drive the company forward and compete in our data-driven economy.
- The need for multiple tools: Many IT departments still need to use multiple tools to meet all of their needs. They have one tool for ETL, another one for visualization, yet another tool for machine learning, and so forth. This becomes an issue because there are many different tools and results that need to be monitored and maintained. An increasing number of companies are looking for a platform that provides seamless integration of these tools so that they don’t need to continuously learn new applications, flip through different screens and reinvent the wheel every time a new need arises within their organization. While newer platforms are making progress in terms of bringing disparate tools together under one platform, there is still quite some distance to cover to help companies interact with their analytics apparatus seamlessly.
Fortunately, the analytics realm is an ever-evolving one
Tellius has introduced a new era in business intelligence by offering a solution, leveraging advanced machine learning algorithms that finds insights automatically while simultaneously letting you explore your information assets using a search-based interface.
Taking a user-centric approach, we developed a platform that addresses chief stumbling blocks to a successful big data adoption to put users in the driving seat of their data. To learn about how Tellius is driving the world of analytics forward, contact us.
Get release updates delivered straight to your inbox.
No spam—we hate it as much as you do!
Related blog posts
Tool and strategies modern teams need to help their companies grow.
FAQ
Lorem ipsum dolor sit amet, consectetur adipiscing elit. Suspendisse varius enim in eros elementum tristique.
Contact