Decision Intelligence vs Business Intelligence: How I Learned to Stop Digging Through Dashboards and Love Insights
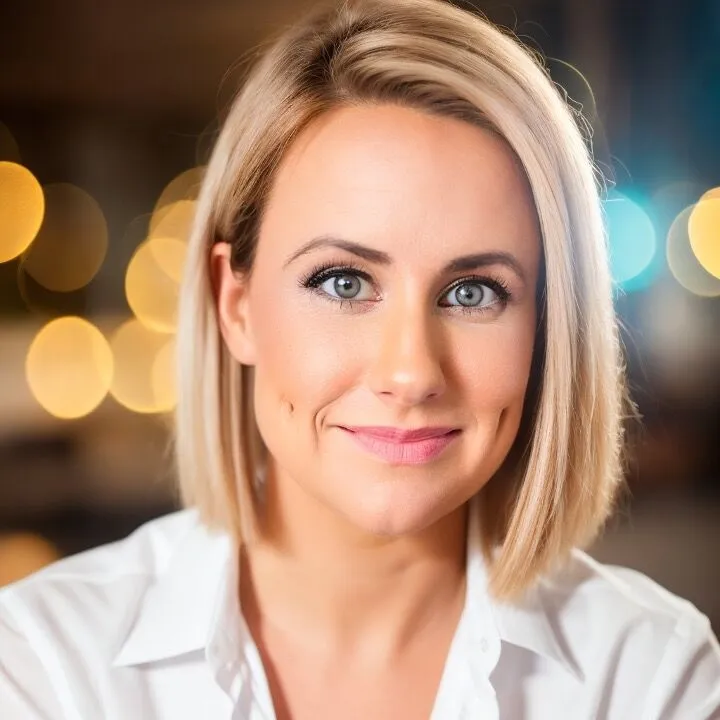

Me after looking at looking at my 50th revenue chart of the day
Data has been collected since ancient history. The Inca people used knotted, colored fiber strings called quipus for collecting data and keeping records — from tax obligations to census records and calendrical information.

Finding use for this data was limited by the power of the human brain for centuries. The brain is a powerful thing, but it can’t scale to comprehend any nuance in millions of data points. An Inca tax collector may have been able to understand at a glance the amount of crops owed from a single cord, but they could not easily add up all of this information in order to determine who was more likely to avoid their tax burden.
Tableau, Qlik and PowerBI (from here on the “Big 3 BI vendors”) advanced the world into modern times by giving tech-savvy analysts the power to visualize data. Freed from the burden of an IT-driven analytics organization, companies from the early 00s have been free to completely integrate data-driven decision making into their business model. Right?
Wrong.
The Big 3 BI vendors did move analytics away from a process with months-long cycle times, helping companies develop independent analytics organizations. Today, developers create dashboards and share with their peers. Some of those dashboard consumers were good at manipulating filters and getting to the information that they need, some are not.
The current analytics process is still not nirvana. The Big 3 have managed to move us from a months-long process to one that is still days and even weeks long at time. BI is focused on an individual question with a static premise — for example, “how much did my gross margin increase this quarter in Florida?”. We will call these “static questions”, defined as one-off questions with limited value in a silo.

Static questions, supported by the current BI infrastructure, can take anywhere from hours to days to answer a simple question. We’ve trained business people to break their question business question down into multiple static questions to support a decision. Why? This are the types of questions legacy BI can handle.
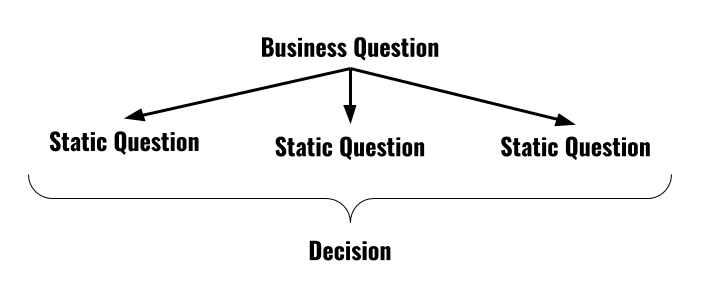
Businesses make hundreds of decisions every day supported by many thousands of iterative static questions. At scale, BI is driving tens of thousands of hours of inefficiency. There is a cost to wasting time, and it isn’t just productivity and time savings — missed opportunities and opportunity costs abound due to slow process.
Inefficient processes not only result in decision taking longer, but they also mean unhappy people. If you’ve ever worked with a stupid software tool or platform, you know that you’d rather be doing anything other than working with that tool. Unhappy people avoid making data driven decision, even when those decisions might yield better results.
Clearly, a new way of thinking is necessary.
Enter decision intelligence
Organizations around the world are modernizing the way they think about making decisions by introducing decision intelligence. Instead of asking “what happened to my web traffic in New Jersey last month”, decision intelligence supports questions like “why are my customers not buying my product”?
Instead of breaking business questions down into multiple static questions that map back to specific BI tools and datasets, decision intelligence automatically provides relevant, actionable insights into your data.
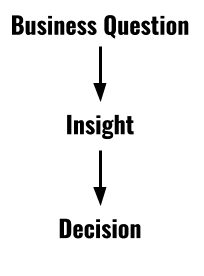
How does it work?
Decision intelligence provides the right piece of information at the right time, in the context of the question being asked. This is done utilizing a few new technologies: natural language search and machine learning models.
Legacy BI applications were architected 20 years ago. They are built around older data structures that pre-aggregated results, didn’t support machine learning and featured pre-defined drill paths and static dashboards.
Decision intelligence uses a modern data architecture and massively parallel processing to take all of a company’s data into account. This provides deep insights taking every piece of available information into account — not the case with BI today.
Another differentiator for DI is the user experience that it supports. I was on a call with a customer and another vendor yesterday, and that vendor was demonstrating their “powerful analytics technology”. It looked like this:

Compare this to the experience of decision intelligence: natural language based, allowing for a flexible data journey wherever the user wants to go. The output of decision intelligence tools is also markedly different. Instead of giving the user hundreds of dashboards and letting them play “find the answer”, DI tools aim to expose insights immediately.
Insights are intuitive, simple, easy to understand answers that are summarized neatly on a single page. Real insights allow for a user to ask a business question, without having to break it down into multiple static questions that are compatible with their legacy BI tool, and get an answer that they can immediately appreciate and act on.
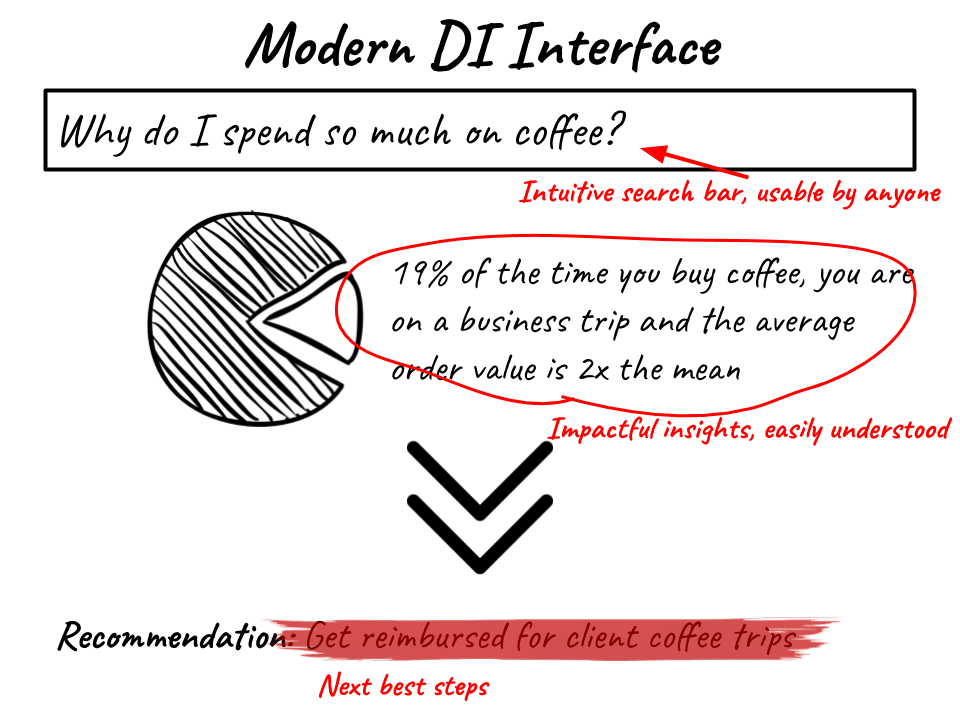
For those of you out there still on the fence, let me break it down with an analogy. 1,000 years ago it took weeks to travel a significant distance. 100 years ago it took days. Today, you can step on a plane and go anywhere on the world in < 24 hours.
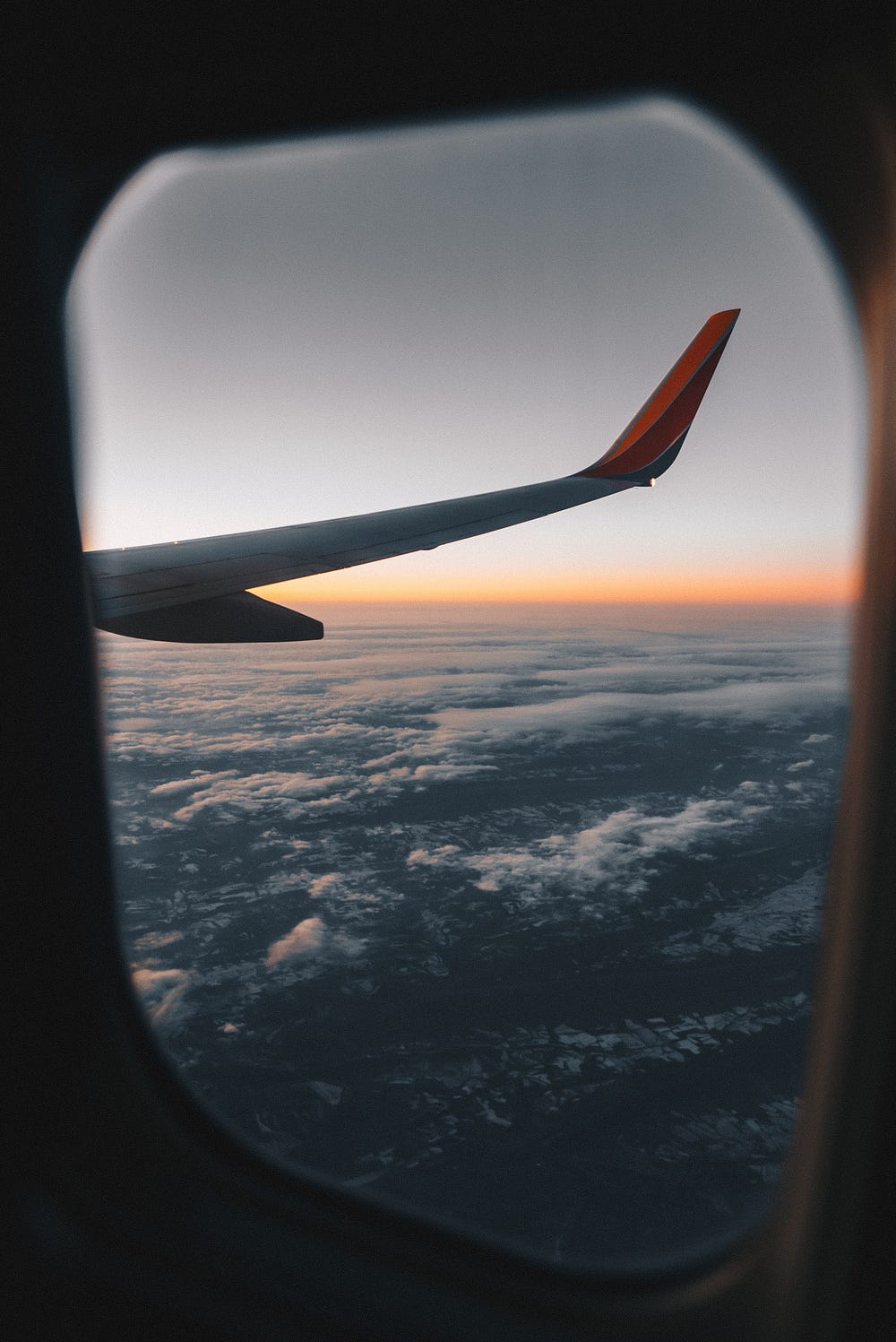
Efficiency and affordability of travel has opened up doors that were never possible for million of people and, along with food production, has helped the world to advance to where it is today. Time once spent traveling is now spent exploring, learning, working and growing. Where travel was once a barrier to growth, it is now an enabler.
Business intelligence is holding you back from experiencing that same growth within your own career. The question is not
why should we be using decision intelligence?
In fact, the question you should be asking is
why are we not using decision intelligence?
Get release updates delivered straight to your inbox.
No spam—we hate it as much as you do!
Related blog posts
Tool and strategies modern teams need to help their companies grow.